Systems Thinking and AI for Evidence-Based Prevention Policy
Why focus on this topic?
Systems thinking and complexity science (STCS) and artificial intelligence (AI) are rapidly evolving fields with emerging potential for application in population health and policy design and evaluation. STCS is broadly characterised by the idea that real-world phenomena exist within systems composed of dynamic actors, including people, organizations and other structures, which evolve in response to each other and their contexts. The field of AI also encompasses a range of methods and is characterised by the use of computer systems to interpret and learn from data.
How is this research done?
Given the complex causal origins of disease, and the dynamic landscapes in which policies designed to tackle disease are made and unfold, this work aims to explore the potential uses of STCS and AI in developing and evaluating effective policy solutions. With this in mind, we are conducting primary research using these methods, as well as leading and supporting the development of practical guidance and tools to facilitate the use of these methods by practitioners working in disease prevention. Our work around STCS and AI methods covers topics including food systems, the prevention of both non-communicable and infectious diseases, policymaking, and evaluation.
What do we expect to find and why will it matter?
The findings from our review work will provide researchers and policymakers with the range of STCS methods that have been applied in NCD prevention policy, presenting insights into their advantages and limitations, as well as barriers and facilitators to their use. Developing guidance based on the review to support systems thinking approaches in practice is also underway. STCS methods have the potential to generate tailored and dynamic evidence, adding robustness to evidence-informed policymaking, but must be accessible to policy stakeholders and have strong stakeholder ownership.
Sources
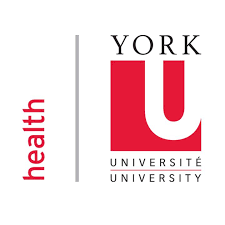
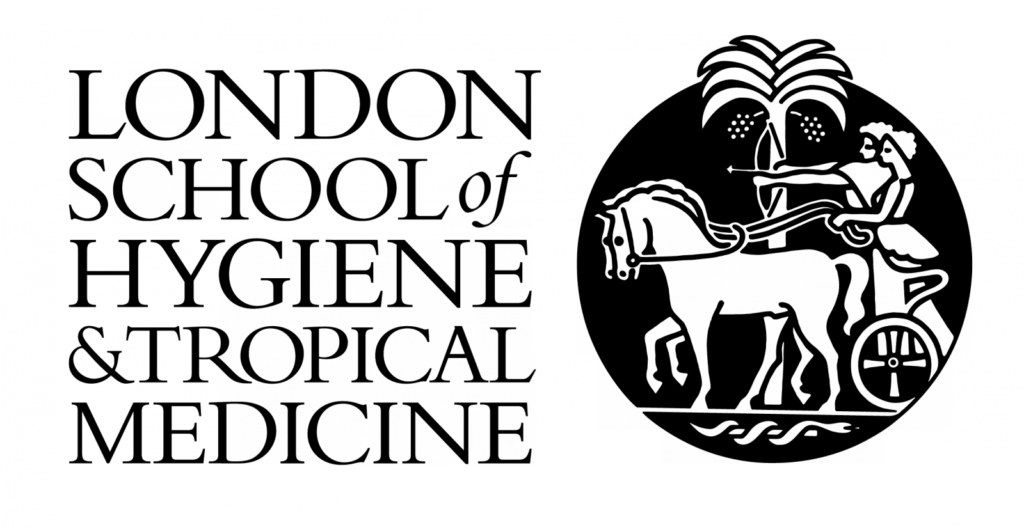
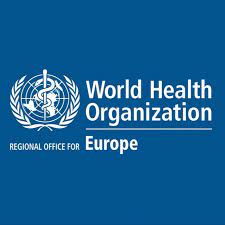